In the world of research and data analysis, independent variables play a crucial role. Whether you’re conducting an experiment, analyzing data, or making business decisions, understanding how independent variables influence outcomes can significantly improve your interpretations. So, what exactly are independent variables, and how do they impact your research? we’ll break it down and provide practical tips to help you better interpret your data.
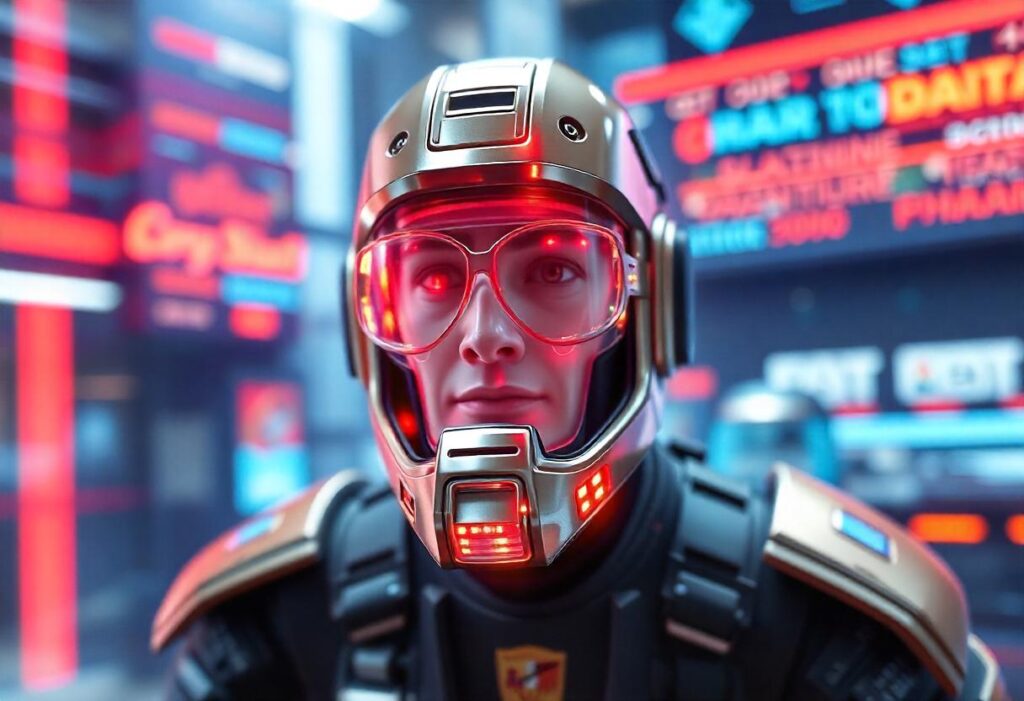
What Are Independent Variables?
An independent variable is the variable that a researcher changes or controls in an experiment to test its effects on other variables. Think of it as the “cause” that influences the “effect.” For example, in a study on how different fertilizers affect plant growth, the type of fertilizer would be the independent variable. It’s the factor that’s being manipulated to observe the results.
Independent vs. Dependent Variables
To fully grasp the concept of an independent variable, you need to understand its counterpart: the dependent variable. While the independent variable is what you change, the dependent variable is what you measure. In our plant example, plant growth (height, health, etc.) would be the dependent variable because it’s affected by the type of fertilizer used.
Types of Independent Variables
Manipulated Independent Variables
These are the variables that the researcher intentionally changes in an experiment. For instance, changing the temperature in a lab to see how it affects chemical reactions involves manipulating the independent variable (temperature).
Subject Variables
Subject variables refer to characteristics inherent to the subjects being studied that can’t be manipulated, such as age, gender, or socioeconomic status. In some studies, these are treated as independent variables even though they aren’t directly controlled by the researcher.
The Role of Independent Variables in Experiments
Independent variables are at the core of experimental research. By controlling the independent variable, researchers can observe how it affects the dependent variable. This helps them understand cause-and-effect relationships, making independent variables essential for hypothesis testing.
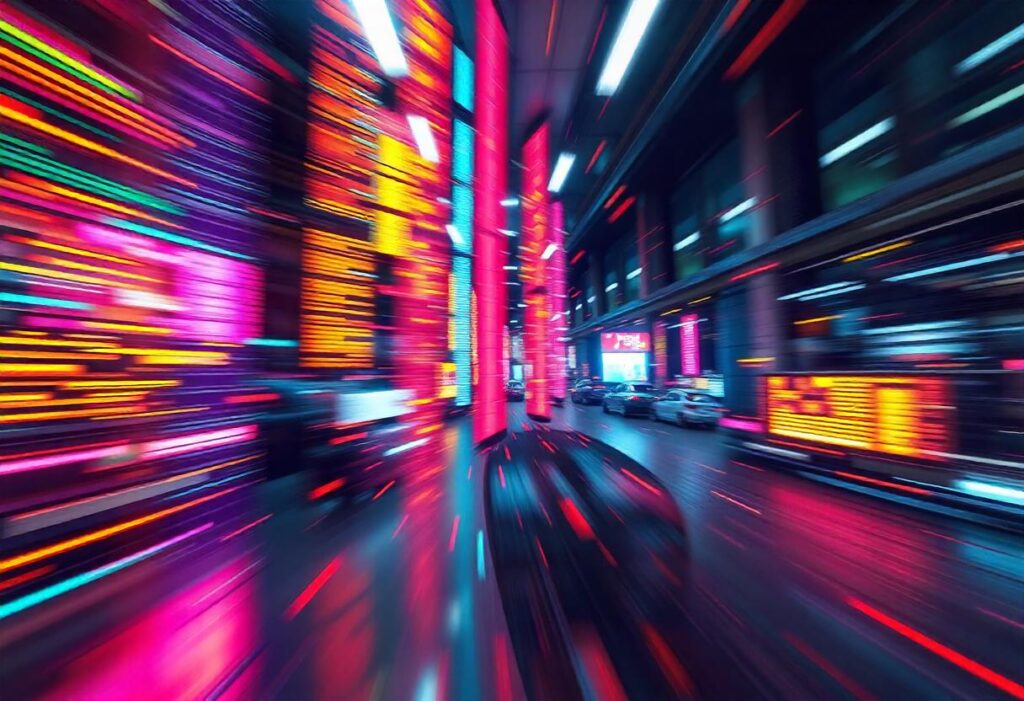
Examples of Independent Variables in Different Fields
- In Medicine: The dosage of a drug given to patients is an independent variable, with the dependent variable being the patients’ responses or side effects.
- In Education: The type of teaching method used (e.g., lectures vs. interactive learning) can be the independent variable, while student performance is the dependent variable.
- In Marketing: Changing the price of a product can be an independent variable, with the sales volume as the dependent variable.
Identifying Independent Variables in Studies
In any given study, identifying the independent variable is crucial for understanding the experiment’s design. Researchers must ask themselves, “What am I changing to observe the effect?” For example, in a study testing the effect of sleep on cognitive performance, the number of hours slept would be the independent variable.
Real-World Examples
- In a clinical trial, the independent variable might be the treatment being tested, such as a new drug, while the outcome (e.g., reduction in symptoms) is the dependent variable.
- In a psychological experiment, the independent variable could be the type of stimulus given to participants, while their reactions would be the dependent variable.
Independent vs. Dependent Variables: A Clear Distinction
While both independent and dependent variables are crucial to research, they serve different purposes. The independent variable is what you manipulate, while the dependent variable is what changes as a result of that manipulation. Understanding this distinction ensures that you can properly interpret the results of an experiment.
How They Work Together
In any study, the independent and dependent variables work hand-in-hand. The independent variable provides the cause, while the dependent variable reflects the effect, allowing researchers to draw conclusions about the relationship between the two.
Why Independent Variables Are Crucial for Data Interpretation
When it comes to interpreting data, the independent variable is often the key to unlocking the “why” behind your findings. Misidentifying or misunderstanding the independent variable can lead to faulty conclusions, which is why it’s critical to define and control it correctly.
Impact on Results
A poorly chosen or defined independent variable can skew results or lead to ambiguous conclusions. For example, failing to control for a variable like time of day in a study on productivity could confound the results, making it unclear whether the productivity was influenced by the time or another factor.
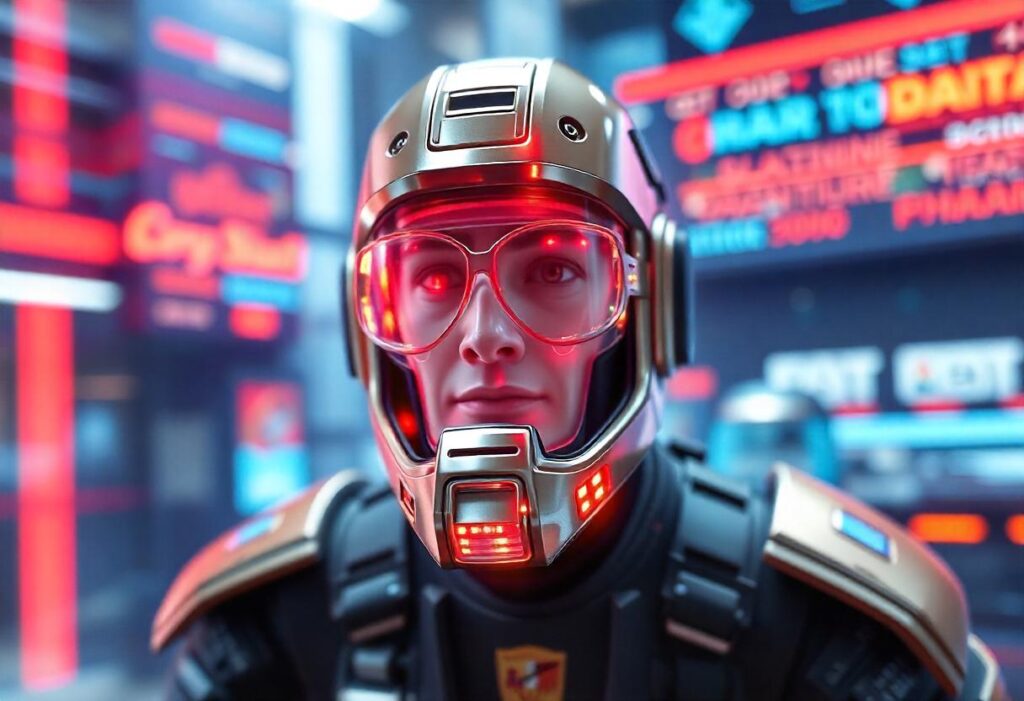
How to Manipulate Independent Variables for Better Results
To ensure reliable and valid results, researchers often manipulate independent variables in controlled ways. This can involve altering the dosage of a drug, adjusting environmental conditions, or introducing different stimuli to participants.
Experimental Control and Variation
Maintaining control over your independent variables is key. Researchers must also ensure that variations introduced by the independent variable are consistent and measurable to draw clear conclusions.
Challenges in Working with Independent Variables
Confounding Variables
Confounding variables are factors that can obscure the relationship between the independent and dependent variables. For instance, if you’re studying the effect of exercise on weight loss but fail to account for participants’ diets, diet becomes a confounding variable.
Dealing with Bias
Bias can creep in if independent variables are not carefully controlled. Ensuring that other factors don’t influence the outcome is essential for accurate data interpretation.
The Role of Independent Variables in Correlation vs. Causation
One common pitfall in data interpretation is confusing correlation with causation. Just because two variables are related doesn’t mean one caused the other. Independent variables help establish causality, but it’s vital to control for confounding factors to avoid false conclusions.
Common Mistakes in Interpretation
It’s easy to mistake a correlation for causality. For example, if ice cream sales and drowning incidents both increase in the summer, it doesn’t mean ice cream causes drowning. The true independent variable here could be the rise in temperature.
The Importance of Properly Defining Independent Vari–ables
To avoid errors, researchers must clearly define their independent vari-ables from the start. This ensures that the results are attributable to the right factors.
Tips for Setting Up Research
- Clearly state what the independent variable is and how it will be manipulated.
- Ensure that confounding variables are controlled or accounted for.
- Be consistent in the measurement and application of the independent variable.
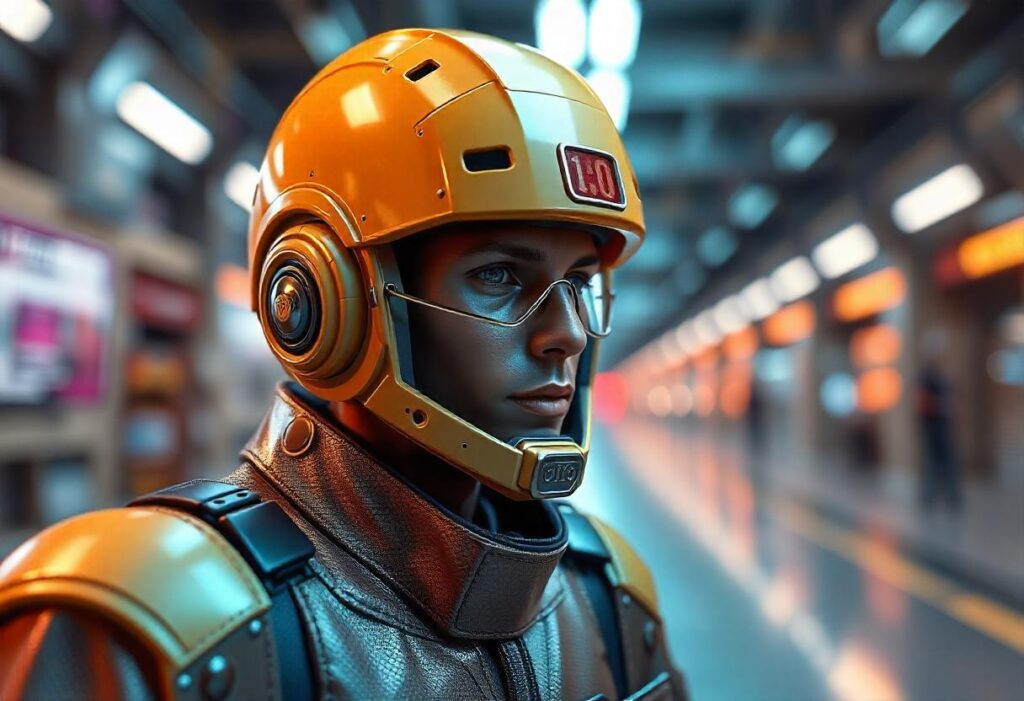
Best Practices for Using Independent Variables in Research
Planning and precision are key when working with independent vari-ables. Properly defining and controlling them allows researchers to generate reliable, valid, and replicable results.
Ensuring Validity and Reliability
To guarantee that your findings are accurate, ensure that your independent variable is both valid (it measures what it’s supposed to) and reliable (it produces consistent results).
Interpreting Data with Multiple Independent Vari-ables
Some experiments use more than one independent variable, which can complicate data interpretation. For instance, a study might examine the effects of both temperature and humidity on plant growth. In these cases, researchers use factorial designs to analyze how the variables interact.
Factorial Designs and Analysis
A factorial design allows researchers to study the interaction between multiple independent vari-ables and how they jointly affect the dependent variable. This approach is common in fields like psychology and biology.
The Influence of Independent Variables on Statistical Analysis
Independent vari-ables are a key factor in statistical tests. Different types of analyses are used depending on the number and type of independent vari-ables in a study.
Regression Analysis and ANOVA
Regression analysis examines the relationship between one or more independent vari-ables and the dependent variable. ANOVA (Analysis of Variance) is used when comparing the effects of different levels of an independent variable across groups.
Conclusion
Independent variables are the foundation of any research experiment. By carefully defining and controlling them, researchers can draw meaningful conclusions and make accurate predictions. Whether you’re in the lab or analyzing data, understanding how independent vari-ables work will help you interpret your results more effectively.
FAQs
1. What is an example of an independent variable in an experiment?
An independent variable is something the researcher changes or manipulates, such as temperature, to observe its effect on another variable.
2. Can a study have more than one independent variable?
Yes, many studies use multiple independent vari-ables to explore more complex relationships between factors.
3. How do you control for confounding variables?
By carefully designing the experiment and controlling for factors that could influence the results, such as using a randomized control group.
4. Why are independent vari-ables important in data analysis?
They help researchers establish cause-and-effect relationships, making it easier to interpret the results of a study.
5. What’s the difference between correlation and causation?
Correlation is when two variables are related, but causation implies that one variable directly affects the other.